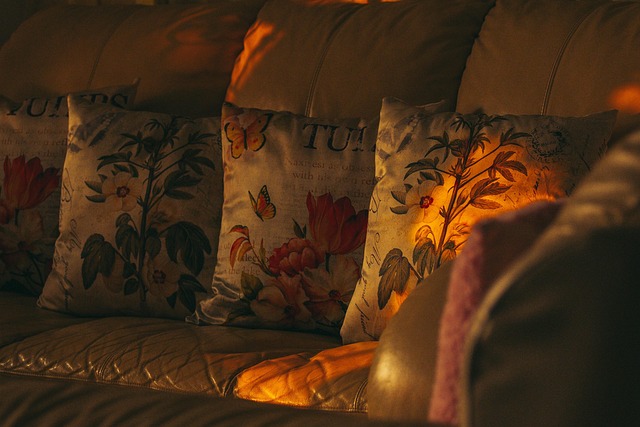
Causal Effect
Understanding Causal Effect
The concept of causal effect is fundamental in various fields, including statistics, social sciences, and health research. It refers to the impact that one variable (the cause) has on another variable (the effect). Understanding causal effects is crucial for making informed decisions based on empirical data, particularly in experimental and observational studies.
Theoretical Foundations
The modern understanding of causal inference is largely attributed to the work of statisticians such as Donald Rubin and Paul Rosenbaum, who developed the Rubin-Rosenbaum-Holland (RRH) theory of causal inference. This theory builds upon earlier experimental designs established by Ronald Fisher and others, emphasizing the importance of controlled experiments in determining causal relationships.
Defining Causal Effect
A causal effect can be defined as the difference in outcomes that an individual would experience under two different conditions. For instance, if a child receives treatment E, the outcome can be observed. Conversely, if the same child receives treatment C, a different outcome is expected. The causal effect is quantified as:
Treatment Effect = Outcome under E - Outcome under C
This formula illustrates that the causal effect is essentially a comparison between the outcomes of two different scenarios for the same subject.
Importance of Randomization
Randomization plays a critical role in establishing causal effects. By randomly assigning subjects to treatment and control groups, researchers can minimize the influence of confounding variables—factors that may affect the outcome but are not of primary interest. This random assignment ensures that the groups are comparable, thereby allowing for a more accurate estimation of the causal effect.
Types of Causal Effects
Causal effects can be categorized into several types, including:
- Average Treatment Effect (ATE): This refers to the average effect of a treatment across a population.
- Conditional Average Treatment Effect (CATE): This measures the treatment effect for specific subgroups within the population.
- Local Average Treatment Effect (LATE): This focuses on individuals who are induced to change their treatment status due to the intervention.
Challenges in Causal Inference
Despite its importance, establishing causal effects is fraught with challenges. One major issue is the presence of confounding variables, which can obscure the true relationship between the cause and effect. Additionally, ethical considerations often limit the ability to conduct randomized controlled trials, particularly in fields such as medicine and social policy.
Another challenge is the potential for selection bias, where the individuals who receive a treatment differ systematically from those who do not. This bias can lead to inaccurate estimates of causal effects if not properly addressed.
Methods for Causal Inference
Researchers employ various methods to infer causality, including:
- Randomized Controlled Trials (RCTs): Considered the gold standard for causal inference, RCTs involve random assignment to treatment and control groups.
- Observational Studies: When RCTs are not feasible, researchers may use observational data, employing statistical techniques to control for confounding variables.
- Propensity Score Matching: This method involves matching individuals in treatment and control groups based on their likelihood of receiving treatment, thereby reducing selection bias.
Applications of Causal Effect
The concept of causal effect is widely applied across various domains:
- Health Research: Understanding the causal effects of treatments on patient outcomes is essential for developing effective medical interventions.
- Social Sciences: Researchers analyze causal relationships to inform policy decisions and understand social phenomena.
- Economics: Economists study causal effects to evaluate the impact of policies and programs on economic outcomes.
Conclusion
In summary, the concept of causal effect is a cornerstone of empirical research across multiple disciplines. By understanding and accurately measuring causal effects, researchers can draw meaningful conclusions that inform practice and policy. The ongoing development of methodologies for causal inference continues to enhance the ability to discern true causal relationships, thereby contributing to the advancement of knowledge in various fields.
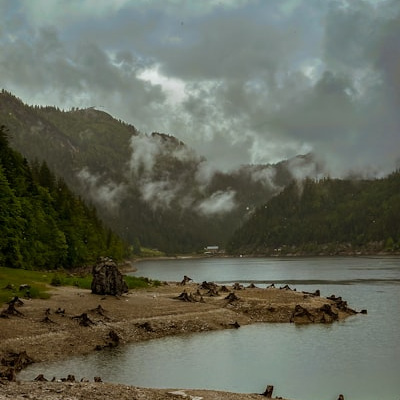
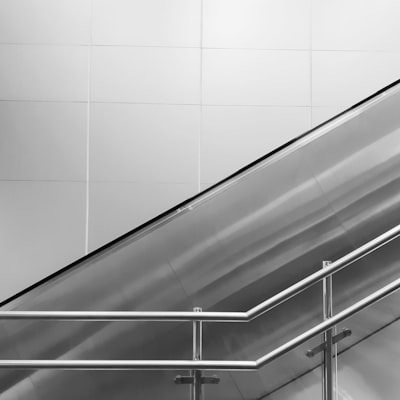