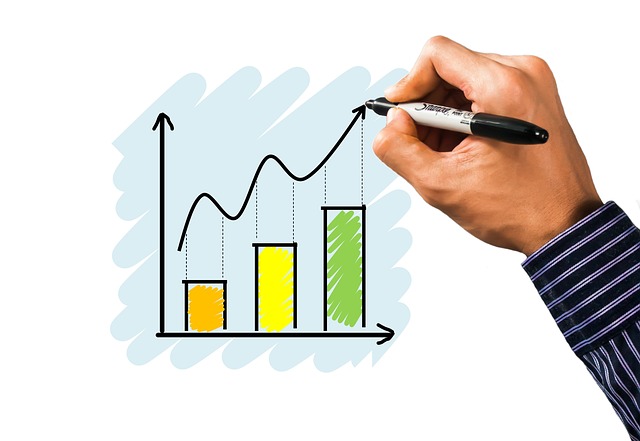
Statistical Models
Understanding Statistical Models
Statistical models are fundamental tools in data analysis, providing a structured way to understand and interpret data. They serve as a framework for making inferences about populations based on sample data. This article will explore what statistical models are, their types, and their applications in various fields.
What is a Statistical Model?
A statistical model can be defined as a mathematical representation of observed data. It comprises a set of assumptions about the underlying processes that generate the data. These assumptions allow statisticians to calculate the probabilities of various outcomes, making it possible to draw conclusions and make predictions.
Types of Statistical Models
Statistical models can be broadly categorized into two main types: parametric and non-parametric models.
- Parametric Models: These models assume a specific form for the distribution of the data. For example, a common parametric model is the normal distribution, which is defined by its mean and standard deviation. Parametric models are often easier to work with because they reduce the complexity of the data to a few parameters.
- Non-parametric Models: Unlike parametric models, non-parametric models do not assume a specific distribution. They are more flexible and can adapt to a wider range of data shapes. Examples include kernel density estimation and certain types of regression models.
Applications of Statistical Models
Statistical models are widely used across various fields, including:
- Economics: Economists use statistical models to analyze economic trends, forecast future economic conditions, and evaluate the impact of policy changes.
- Healthcare: In the medical field, statistical models help researchers assess the effectiveness of treatments, understand disease patterns, and predict patient outcomes.
- Social Sciences: Social scientists employ statistical models to study human behavior, relationships, and societal trends, allowing for a deeper understanding of complex social phenomena.
- Machine Learning: Many machine learning algorithms are based on statistical models, enabling computers to learn from data and make predictions or decisions.
Building a Statistical Model
Creating a statistical model typically involves several key steps:
- Define the Problem: Clearly outline the question or hypothesis you wish to investigate.
- Collect Data: Gather relevant data that will inform your model. This data can come from experiments, surveys, or existing databases.
- Choose a Model: Select an appropriate statistical model based on the nature of your data and the assumptions you are willing to make.
- Fit the Model: Use statistical techniques to estimate the parameters of your model based on the collected data.
- Validate the Model: Assess the model's performance by checking its predictions against actual outcomes. This step is crucial to ensure the model is reliable.
Challenges in Statistical Modeling
While statistical models are powerful, they come with challenges. One major issue is the assumption of the model. If the underlying assumptions do not hold true, the model's predictions can be misleading. Additionally, overfitting—where a model is too complex and captures noise rather than the underlying pattern—can lead to poor generalization to new data.
Conclusion
Statistical models are essential tools for understanding and interpreting data across various disciplines. By providing a structured approach to data analysis, they enable researchers and analysts to make informed decisions based on empirical evidence. As data continues to grow in importance, the role of statistical models will only become more significant.
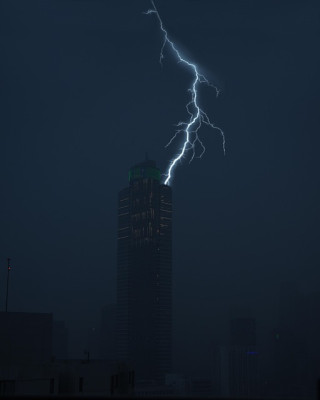